How not to have a biased AI model?
Navigating the challenges posed by technology's increasing centrality requires us to address the biases present in GPT and LLM especially as these models are not effectively trained on non-western countries. Do read on as we unpack this here from a behavioural perspective.
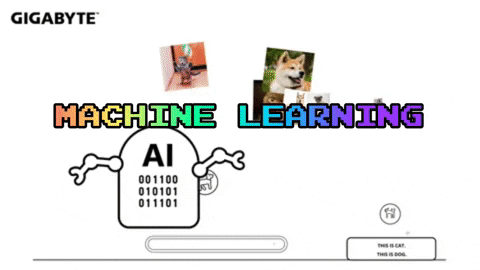
Abstract
Navigating the challenges posed by technology's increasing centrality requires us to address the biases present in Generative Pre-trained Transformers (GPT) and Large Language Models (LLM) especially as these models are not effectively trained on non-western countries. Do read on as we unpack this here from a behavioural perspective.
Mind the Machine
Language Models, including Large Language Models(LLM), are Artificial Intelligence(AI) programs that use statistical probabilities to understand how words are related to each other in a language. They can predict what word is likely to come next in a sentence based on the words that came before it. LLMs are trained on vast amounts of data, including books, websites, and social media posts, to recognize patterns in language and generate new text that is similar in style and content to the text they were trained on.GPT, or Generative Pre-trained Transformer, a specific type of LLM, is an intelligent language tool that uses vast amounts of text to predict the next word in a sentence based on context.
It was developed by OpenAI, a research organization focused on advancing artificial intelligence in a safe and beneficial way. GPT has undergone several iterations since its first release in 2018, with each version becoming more advanced and capable than the last. GPT can be adapted for specific tasks with minimal training, and examples include ChatGPT, Google Bard, Bing AI, and Jukebox. Unlike traditional algorithms that are explicitly programmed for specific tasks, GPT uses unsupervised learning to train on massive amounts of data.The integration of AI into our daily lives is happening at an accelerating pace, and it's impacting us in ways we may not fully understand. On one hand, AI offers significant benefits, such as increased efficiency and productivity. On the other hand, it also raises concerns regarding the ethical and social implications of its usage. In today's digital age, more and more people are both using and creating goods or services at the same time. This is known as being a prosumer, which has become increasingly relevant due to social media platforms and other online services where individuals often leave behind their data while engaging with content. However, there are concerns about about the extent to which companies can use AI, to gather personal data. This raises questions about who owns this information and whether profit-driven businesses might take advantage of prosumers by exploiting their private details.
Unpacking Bias
Bias is a complex and often misunderstood concept that can have far-reaching implications for language learning models. While biases in AI models can be negative and perpetuate harmful stereotypes, there is a growing need to question how we think about bias and look at it more flexibly. At its core, bias refers to a tendency towards a specific direction or perspective. In language models like GPT, bias can manifest as systematic misrepresentations, attribution errors, or factual distortions that favour certain groups or ideas.
This can lead to predictions and decisions influenced by pre-existing prejudices and assumptions. Timnit Gebru, former AI Ethics researcher at Google, highlighted the potential dangers of bias in LLMs as they may pick up on societal prejudices within their training data resulting in biased predictions and decisions.Basically are two types of bias - inductive and cognitive - that can impact how these models function. Inductive biases arise when an algorithm tries to make sense of limited data by making assumptions based on what it has seen before, which can lead to errors if those initial assumptions turn out to be incorrect or incomplete. Cognitive biases, such as confirmation bias or availability bias, can influence how humans interpret the outputs of AI systems, leading to a feedback loop where humans reinforce the biases in the system.The impact of biases in AI algorithms is exemplified by the case of COMPAS is a US criminal justice algorithm that assesses offender recidivism risk and recommends sentences.
ProPublica's study discovered bias against black defendants, with the algorithm predicting more future crimes for them than white defendants with similar records. Judges still relied on the recommendations due to confirmation bias, interpreting information in ways that confirmed their existing beliefs. The study found judges overruled release recommendations for black defendants but followed them when recommended for whites.However, it is important to acknowledge that not all biases are negative. In some contexts, biases can be useful in developing effective AI models. For instance, incorporating behavioural biases that reflect how humans typically behave in certain situations can lead to more natural and effective AI interactions.Reducing bias in language models is challenging due to the complex nature of language and culture. Language is a reflection of society, and as such, it contains biases, stereotypes, and assumptions that are deeply ingrained in language structures and expressions. Furthermore, cultural values can differ substantially across communities or regions, further compounding these issues when trying to establish uniform norms for AI algorithms.Algorithmic decision-making, especially with LLMs, must prioritize fairness to avoid negative impact. However, determining what is fair can be challenging due to various contextual and subjective factors.
The Brookings report has shed light on the need for AI strategies that consider cultural and societal differences outside of Western, Educated, Industrialized, Rich and Democratic (WEIRD) settings. To ensure fairness, it is essential to involve diverse communities in the development process, use inclusive datasets, and regularly evaluate outcomes for potential biases. However, it is important to note that these efforts must be ongoing as biases may emerge over time.To reduce the harmful effects of bias and create AI models that serve diverse communities, it is necessary to approach bias with flexibility and continuously evaluate and improve AI models. This entails collaborating with diverse communities and engaging in ongoing discussions to define fairness, transparency, and equity in the context of language learning models. Although these AI models may constrain certain identities and behaviours, their potential applications are extensive enough to transform various areas of society positively.
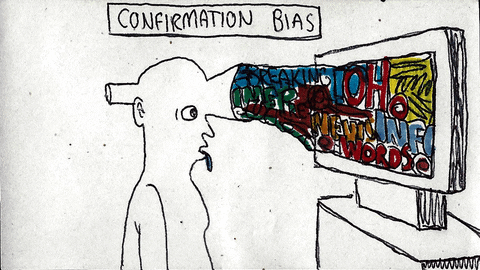
Written by Farheen, Reviewed by Aurko